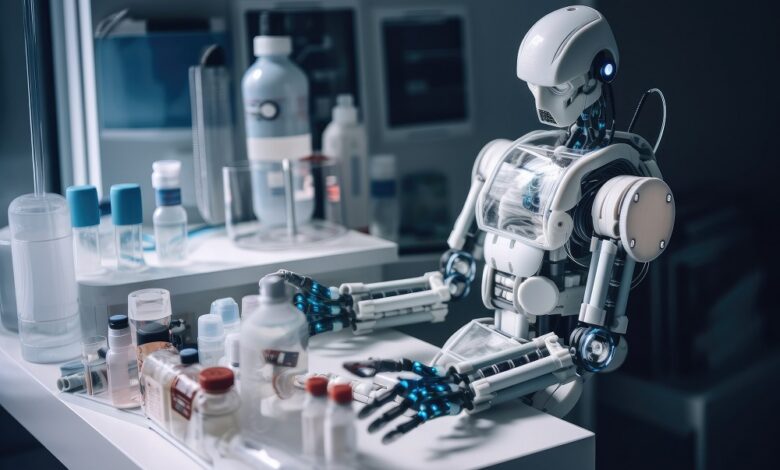
In 2019, the World Health Organization (WHO) declared antimicrobial resistance (AMR) as one of the top 10 threats to global health. AMR is the result of a pathogen-drug combination, where a bacterium, virus or other pathogenic microorganism that causes a disease gains resistance to drugs commonly used to treat the condition.
One pathogen-drug combination of particular concern to health authorities is methicillin-resistant Staphylococcus aureus (MRSA). This pathogen-drug combination was found to be the most deadliest globally in 2019, with more than 121,000 deaths attributed to MRSA in that year. Currently there are no vaccines available for MRSA, and nothing in the near pipeline either.
It is against this background that the recent study by researchers at the Massachusetts Institute of Technology (MIT) in the United States gains salience. Using a type of artificial intelligence (AI) known as deep learning (DL), the researchers were able to discover a class of compounds that can kill MRSA. The compounds could not only destroy the pathogen, they could do so with low toxicity to neighboring cells, making them a potential candidate for drug development.
The MIT study showed that these compounds could kill MRSA grown in a lab dish and in two mouse models infected with MRSA. A major novelty of the study was that the researchers were also able to understand the types of information that the AI model used to select compounds with antibiotic potency. This knowledge could help researchers to design additional drugs that might work even better than the one identified by the MIT team, or even come up with other drugs to fight AMR.
“Our study provides a framework that is time-efficient, resource-efficient, and mechanistically insightful, from a chemical-structure standpoint, in ways that were not available before,” said the MIT researchers leading the Antibiotics–AI Project at MIT. The team is now aiming to discover new classes of antibiotics against seven types of deadly bacteria over the next seven years using AI assistance.
Over the past several years, the researchers have been using the deep learning technique — a method that evolved from the basic type of machine-learning (ML) in artificial intelligence (AI). The method, which draws inspiration from the way the human brain works, can process data to recognize complex patterns in pictures, text, sounds, and other forms of data to provide accurate insights and predictions.
Deep learning (DL) models can be trained to examine chemical compounds and identify chemical structures that are associated with antimicrobial activity. These models can then sift through millions of other compounds, generating predictions of which some may have strong antimicrobial activity. Work by the MIT team has so far yielded potential drugs against several drug-resistant bacteria, including Acinetobacter baumannii, a common bacterium found in hospital environments.
The one limitation to this approach of DL is that the models are ‘black boxes’, meaning that there was no way for researchers to understand what features the model based its predictions on. The researchers at MIT also used their study to try to open this ‘black box’. To do this, the team first trained a deep learning model to test around 39,000 compounds for antibiotic activity against MRSA, and then fed this data, plus information on the chemical structures of the compounds, back into the model.
“You can represent basically any molecule as a chemical structure, and also you tell the model if that chemical structure is antibacterial or not,” Wong says. “The model is trained on many examples like this. If you then give it any new molecule, a new arrangement of atoms and bonds, it can tell you a probability that that compound is predicted to be antibacterial.”
To figure out how the model was making its predictions, the researchers then adapted an algorithm known as Monte Carlo tree search, which has been used to help make other deep learning models. This search algorithm allowed the model to generate not only an estimate of each molecule’s antimicrobial activity, but also a prediction for which substructures of the molecule likely accounted for that activity.
To further narrow down the pool of candidate drugs, the researchers trained three additional deep learning models to predict whether the compounds were toxic to three different types of human cells. By combining this information with the predictions of antimicrobial activity, the researchers discovered compounds that could kill microbes while having minimal adverse effects on the human body.
Using this collection of models, the researchers screened about 12 million compounds, all of which are commercially available. From this collection, the models identified compounds from five different classes, based on chemical substructures within the molecules, that were predicted to be active against MRSA.
The researchers then tested the identified compounds against MRSA grown in a lab dish, which allowed them to identify two, from the same class of compounds, that appeared to be very promising antibiotic candidates. In tests in two mouse models, one of MRSA skin infection and one of MRSA systemic infection, each of those compounds reduced the MRSA population by a factor of 10.
A non-profit spin-off started by members of the MIT team now plans to do more detailed analysis of the chemical properties and potential clinical use of these compounds. By leveraging similar AI processes based on chemical substructures, the researchers hope to design compounds from scratch, and to adopt this approach out of the box to discover new classes of antibiotics against different pathogens.